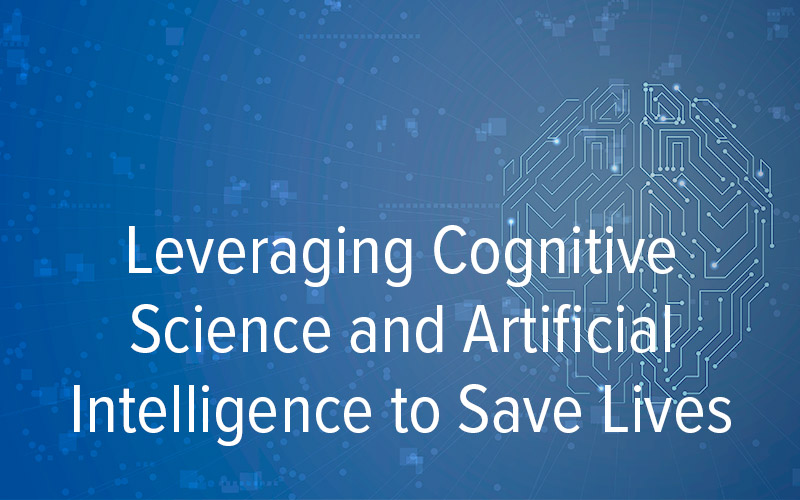
Medical error is the third-leading cause of death in the United States, just behind heart disease and cancer. Implicated in half of these cases of preventable harm, is the confidently held misinformation in the minds of clinicians.
A recent article published by Springer Nature and presented by Amplifire’s Sr. Director of Research and Analytics, Matt Hays, Ph.D, at AIED 2019, shares how an adaptive learning platform harnesses artificial intelligence and the principles of cognitive psychology to find and fix knowledge gaps among clinicians to reduce medical errors.
Artificial intelligence (AI) is widely used in education, largely through intelligent tutoring systems (ITSs). AI is the future, but related findings from cognitive psychology and other learning sciences have gained less traction in ITS research and the classroom. One reason may be that some cognitive phenomena are counterintuitive; how learners, teachers, and even researchers think learning should work is not always how it actually works.5 For example, the testing effect is the finding that retrieving information from memory is much more powerful than being re-exposed to the information (e.g., by re-reading; 6). But classrooms in 2019 still rely heavily on watching videos, sitting through lectures, and reading chapters. Even ITSs often use testing exclusively for assessment purposes (although there are exceptions, e.g., 7).
Amplifire’s adaptive learning platform is built on principles of cognitive science that are proven to promote learning and long-term memory retention. The platform uses AI to determine how best to leverage many of those principles in real time.
AI-Directed Cognitive Science
Amplifire optimizes learning by adapting to each individual’s knowledge base. It uses AI to determine if and when learners need additional learning, provide corrective and metacognitive feedback, and guide learners towards mastery.
The platform begins by asking questions in a variety of formats (multiple-choice, select-all, matching, interactive). This approach is beneficial even if the learner couldn’t possibly know the correct answers.8, 9 Attempting to answer questions is perhaps the most powerful way to gain knowledge and skills,6 even if the answer attempts are incorrect. 10
When responding to questions in Amplifire, learners indicate their confidence in their responses, making them consider the question more carefully 11 and improving their memory for the material 12. This cognitive benefit only occurs when answers and confidence are considered simultaneously,13 a process Amplifire has patented. Learners in Amplifire click an answer once to indicate partial confidence or twice to indicate certainty. They can also click “I don’t know yet.”
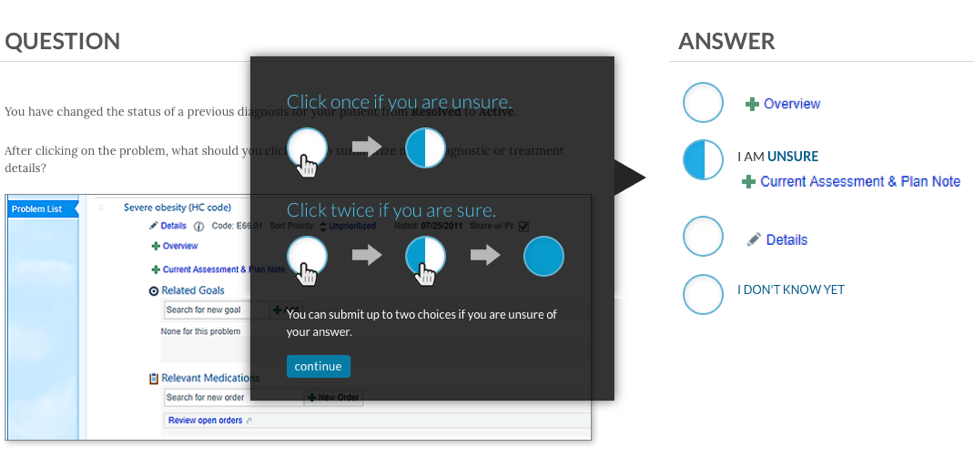
After submitting a response, learners receive immediate feedback on whether their response was correct. Metacognitive feedback guides learners to understand whether they have been under- or overconfident.14 Later they will receive corrective feedback if they answered incorrectly.
Amplifire’s AI also determines whether and when to provide self-regulatory feedback, which is focused on correcting learner behavior in the platform. For example, a learner might be told to “make sure to read the question carefully” if they answer in less time than it would take to read the question.
Corrective feedback for a given item is provided after a delay, which enhances learning.15 Amplifire’s AI optimizes this delay by considering information collected about the learner (e.g., their estimated ability), the content being learned (e.g., the item’s estimated difficulty), and the learner’s response to that particular item (e.g., how long the learner spent reading the prompt). The corrective feedback takes the form of elaborative explanation16 and, when appropriate, worked examples 17. The rationale behind the correct response is provided and the error the learner made is explained (e.g., miscalculation, faulty knowledge, etc.).
Amplifire does not provide corrective feedback after full-confidence correct responses because doing so does not improve retention. 18 Learners’ time is therefore better spent on more productive activities.19 Corrective feedback is, however, provided after partial-confidence correct responses,20 and is especially powerful incases of confidently held misinformation.21
For problems or conceptual questions on which learners were not both fully confident and correct, Amplifire repeatedly tests the learner until its AI has determined that they have reached a mastery state. These repeated attempts profoundly improve the learner’s long-term retention of the material.22 Amplifire’s AI considers learner, content, and response data in order to determine the optimal delay between successive attempts on a concept. This delay harnesses the spacing effect, which is the finding that distributing learning over time is more effective than massing it together.23
Amplifire targets the point in the learner’s forgetting curve where a retrieval attempt is difficult but not impossible.24, 25
Altogether, Amplifire leverages AI and cognitive science to optimize the learner’s time spent mastering the material, promote long-term retention and transfer to related tasks, and maintain learner engagement.
Application and Efficacy in Healthcare
Amplifire has partnered with career-focused online universities, GED providers, and other educational institutions that support non-traditional and underserved student populations. More recently, Amplifire has expanded into healthcare training and formed the Healthcare Alliance.
Medical errors are responsible for more than 250,000 fatalities in the United States annually, making them the third-leading cause of death.26 More than half of all medical errors are attributed to the “cognitive failures” of healthcare professionals.27 Amplifire was used at a large healthcare system to combat the cognitive failures that contribute to two hospital-acquired infections: CLABSI and CAUTI. The healthcare system made no other changes to policies, training, or available resources during this period; all effects were attributed to Amplifire.
Central-Line-Associated Bloodstream Infections (CLABSI)
A central line is a thin tube (catheter) placed into a large vein. Central lines are used to administer nutrition or medication (e.g., drugs for chemotherapy), and to monitor central blood pressure during acute care. When a healthcare provider inadvertently contaminates the equipment or the insertion site, the patient can develop a central-line associated bloodstream infection (CLABSI). The incidence of CLABSI is expressed in terms of the number of infections caused for every 1,000 days that patients had central lines (“CLABSI per 1,000 line-days”).
All central-line-attending nurses at a large healthcare system (N = 3,712) were trained in Amplifire. The results are displayed in the left panel of Fig. 1. In the 28 months before training, there were 1.09 CLABSI per 1,000 line-days. In the seven months after training, there were 0.56 CLABSI per 1,000 line-days—a reduction of 48%. An exact Poisson test indicated a statistically significant reduction in the CLABSI rate after training: p = .00014. Given CLABSI’s mortality rate of 25%, this reduction should save approximately 13 lives per year at this health system.28
Catheter- Associated Urinary-Tract Infections (CAUTI)
A urinary catheter is a thin tube inserted into the bladder via the urethra. An indwelling catheter remains in the urethra and bladder for continuous drainage of urine and monitoring of urine output during acute care. As with central lines, healthcare workers’ mistakes can contaminate the catheter and cause a catheter-associated urinary tract infection (CAUTI). Similar to CLABSI, the incidence of CAUTI is expressed in terms of the number of infections caused for every 1,000 days that patients were catheterized (“CAUTI per 1,000 catheter-days”).
Urinary-catheter-attending nurses (N = 4,512) at the same healthcare system were trained in Amplifire. The results are displayed in the right panel of Fig. 1. In the 28 months before training, there were 1.29 CAUTI per 1,000 catheter-days. In the seven months after training, there were 0.88 CAUTI per 1,000 catheter-days—a reduction of 32%. An exact Poisson test indicated a statistically significant reduction in the CAUTI rate after training: p = .01363.
Although both CLABSI and CAUTI were reliably reduced, the smaller magnitude of the CAUTI reduction may be attributable to two factors. First, only nurses interact with central lines, but both nurses and technicians interact with urinary catheters; part of the caregiver population was not trained on CAUTI. Second, the CAUTI course did not employ any multimedia.29 A revised and improved CAUTI course will be distributed to both nurses and technicians in the coming months.
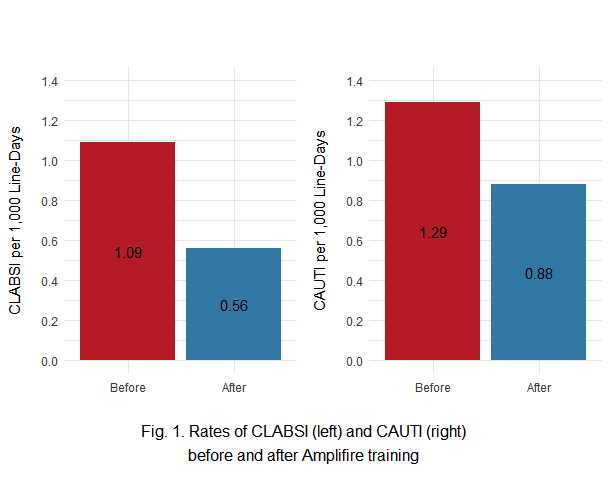
Amplifire relies on principles of cognitive science. By allowing AI to determine how best to leverage many of those principles in real time, Amplifire delivers individually optimized learning in a wide variety of domains (learn more about our role in healthcare, aviation, and higher education). Its test-focused approach improves learners’ ability to retrieve information from memory. Its emphasis on confidence creates an additional dimension of learner introspection and understanding. Its multiple types of scaffolded feedback ensure that difficulty, engagement, and remediation are managed effectively, while also supporting metacognition and self-regulation. Amplifire’s ability to substantially reduce medical error demonstrates the power of cognitive science working hand in hand with AI.
References
1. Kapros, E., Koutsombogera, M. (eds.): Designing for the User Experience in Learning Systems. HCIS, pp. 1–11. Springer, Cham (2018). https://doi.org/10.1007/978-3-319-94794-5
2. Gertner, A., Conati, C., VanLehn, K.: Procedural help in Andes: generating hints using a Bayesian network student model. In: Proceedings of the Fifteenth National Conference on Artificial Intelligence, AAAI, vol. 98, pp. 106–111. The MIT Press, Cambridge (1998)
3. Falmagne, J.-C., Cosyn, E., Doignon, J.-P., Thiéry, N.: The assessment of knowledge, in theory and in practice. In: Missaoui, R., Schmidt, J. (eds.) ICFCA 2006. LNCS (LNAI), vol. 3874, pp. 61–79. Springer, Heidelberg (2006). https://doi.org/10.1007/11671404_4
4. Olney, A.M., et al.: Guru: a computer tutor that models expert human tutors. In: Cerri, S.A., Clancey, W.J., Papadourakis, G., Panourgia, K. (eds.) ITS 2012. LNCS, vol. 7315, pp. 256–261. Springer, Heidelberg (2012). https://doi.org/10.1007/978-3-642-30950-2_32
5. Bjork, R.: Memory and metamemory considerations in the training of human beings. In: Metcalfe, J., Shimamura, A. (eds.) Metacognition: Knowing About Knowing, pp. 185–205. MIT Press, Cambridge (1994)
6. Roediger, H., Karpicke, J.: Test-enhanced learning: taking memory tests improves long-term retention. Psychol. Sci. 17, 249–255 (2006)
7. Bhatnagar, S., Lasry, N., Desmarais, M., Charles, E.: DALITE: asynchronous peer instruction for MOOCs. In: Verbert, K., Sharples, M., Klobučar, T. (eds.) EC-TEL 2016. LNCS, vol. 9891, pp. 505–508. Springer, Cham (2016). https://doi.org/10.1007/978-3-319-45153-4_50
8. Bransford, J., Schwartz, D.: Rethinking transfer: a simple proposal with multiple implications. In: Iran-Nejad, A., Pearson, P. (eds.) Review of Research in Education, vol. 24, pp. 61–100. American Educational Research Association, Washington, DC (1999)
9. Hays, M., Kornell, N., Bjork, R.: When and why a failed test potentiates the effectiveness of subsequent study. J. Exp. Psychol. Learn. Mem. Cogn. 39, 290–296 (2013)
10. Pashler, H., Rohrer, D., Cepeda, N., Carpenter, S.: Enhancing learning and retarding forgetting: choices and consequences. Psychon. Bull. Rev. 14, 187–193 (2007)
11. Bruno, J.: Using MCW-APM test scoring to evaluate economics curricula. J. Econ. Educ. 20 (1), 5–22 (1989)
12. Soderstrom, N., Clark, C., Halamish, V., Bjork, E.: Judgments of learning as memory modifiers. J. Exp. Psychol. Learn. Mem. Cogn. 41, 553–558 (2015)
13. Sparck, E., Bjork, E., Bjork, R.: On the learning benefits of confidence-weighted testing. In: Cognitive Research: Principles and Implications, vol. 1 (2016)
14. Azevedo, R.: Computer environments as metacognitive tools for enhancing learning. Educ. Psychol. 40, 193–197 (2010)
15. Butler, A., Karpicke, J., Roediger, H.: The effect of type and timing of feedback on learning from multiple-choice tests. J. Exp. Psychol. Appl. 13, 273–281 (2007)
16. Shute, V., Hansen, E., Almond, R.: An Assessment for Learning System Called ACED: Designing for Learning Effectiveness and Accessibility. ETS Research Report Series, pp. 1–45 (2007)
17. McLaren, B.M., van Gog, T., Ganoe, C., Yaron, D., Karabinos, M.: Worked examples are more efficient for learning than high-assistance instructional software. In: Conati, C., Heffernan, N., Mitrovic, A., Verdejo, M.F. (eds.) AIED 2015. LNCS (LNAI), vol. 9112, pp. 710–713. Springer, Cham (2015). https://doi.org/10.1007/978-3-319-19773-9_98
18. Pashler, H., Cepeda, N., Wixted, J., Rohrer, D.: When does feedback facilitate learning of words? J. Exp. Psychol. Learn. Mem. Cogn. 31, 3–8 (2005)
19. Hays, M., Kornell, N., Bjork, R.: The costs and benefits of providing feedback during learning. Psychonomic Bulletin and Review, vol. 17, pp. 797-801 (2010).
20. Butler, A.; Karpicke, J., Roediger, H.: Corrective a metacognitive error: Feedback in-creases retention of low-confidence correct responses. Journal of Experimental Psychology: Learning, Memory, and Cognition, vol. 34, pp. 918-928 (2008).
21. Butterfield, B., Metcalfe, J.: Errors committed with high confidence are hyper-corrected. Journal of Experimental Psychology: Learning, Memory, and Cognition, vol. 27, pp. 1491-1494 (2001).
22. Karpicke, J., Roediger, H.: Repeated retrieval during learning is the key to long-term retention. Journal of Memory and Language, vol. 57, pp. 151-162 (2007).
23. Cepeda, N., Pashler, H., Vul, E., Wixted, J., Rohrer, D.: Distributed practice in verbal recall tasks: A review and quantitative synthesis. Psychological Bulletin, vol. 132, pp. 354-380 (2006).
24. Landauer, T., Bjork, R.: Optimal rehearsal patterns and name learning. In: Gruneberg, M., Morris, P., Sykes, R., Practical aspects of memory, pp. 625-632. Academic Press, Lon-don (1978).
25. Hays, M., Darrell, J., Smith, C.: The forgetting curve(s) of 710,870 real-world learners. Poster presented at the American Psychological Association 124th Annual Convention, Denver, CO, USA (2016).
26. Makary, M., Daniel M.: Medical error—the third leading cause of death in the US, BMJ, vol. 353, pp. 2139 (2016).
27. Joint Commission: Patient safety. Joint Commission Online. (2015). https://www.jointcommission.org/issues/article.aspx? Article=jjLkoItVZhkxEyGe4AT5NDyAZaTPkWXc50Ic3pERKGw%3D, last accessed 2019/02/08.
28. CDC: Vital Signs: Central Line–Associated Blood Stream Infections — United States, 2001, 2008, and 2009. In: Morbidity and Mortality Weekly Report (MMWR), vol. 60, pp. 1-6 (2011).
29. Mayer, R.: Using multimedia for e-learning. Journal of Computer Assisted Learning, vol. 33, pp. 403-423 (2017).